Towards the widespread adoption of explainable AI in Banking
With the growth in data which is being generated within the banking and financial sectors the use cases of Artificial Intelligence (AI) in front-office, middle-office and back-office activities in banks is growing steadily, including for fraud detection, risk management, predictive analytics, automation ,and more.
However, there are concerns related to the suitability of the AI models to regulatory frameworks as well as risks related to possible biases in Machine Learning (ML) algorithms which can be due to data quality or when not applying proper business context to the model.
Hence, regulators globally are mandating that financial institutions supply transparent models which can be easily analysed and understood. Many view Explainable AI (XAI) as a critical component in making AI work in heavily-regulated industries like banking and finance.
According toUSA Defence Advanced Research Projects Agency as shown in Figure 1, there are three main approaches to realise XAI. The first approach is termed as deep explanation whose outputs could not be easily analysed or augmented by a lay user.
The second approach is interpretable models which are techniques to learn more structured and interpretable casual models which could apply to statistical models, graphical models or Random Forests. However, even for Logistic Regression or Decision tree models (which are highly accepted as transparent models), using them with a large number of features converts them to black box models.
The third XAI approach is termed model induction which can simply adjust the weights and measures for the inputs to evaluate their effect on the outputs, drawing logical inferences in the process. Other means of model induction interpretability revolve around surrogate or local modelling.
Another facet of explainability relates to rules which not only aid explainability but are also influential in customer relations. aLIME was presented to explain individual predictions with crisp logic IF-Then rules. However the IF-Then crisp anchor model will struggle with variables which do not have clear crisp boundaries, like income (Figure 1a), age, etc and will not be able to handle models with a large number of inputs.
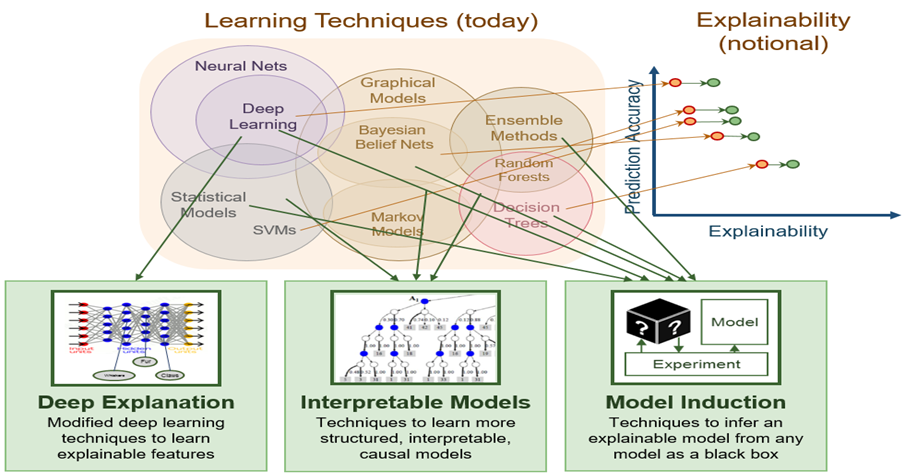
Figure 1. Existing AI techniques- Performance vs Explainability [Courtesy of DARPA].
It seems that offering the user with IF-Then rules which include linguistic labels appears to be an approach which can facilitate the explainability of a model and its outputs. One AI technique which employs IF-Then rules and linguistic labels is the Fuzzy Logic System (FLS) which can model and represent imprecise and uncertain linguistic human concepts. As shown in Figure 2b, by employing the type-1 fuzzy sets in Figure 2b, it can be seen that no sharp boundaries exist between sets and that each value in the x axis can belong to more than one fuzzy set with different membership values. For example, a value of $150,000 belongs now to the “Low” set with a membership value of 0.3 and to the “High” set with a membership value of 0.7. This can mean that if ten people were asked if $150,000 is Low or High income, 7 out of 10 would say “High“, and 3 out of 10 would say “Low“. Furthermore, FLSs employ linguistic IF-THEN rules which enable representing the information in a human-readable form.
Another way to represent linguistic labels is by employing type-2 fuzzy sets as shown in Figure 2c which embeds all the type-1 fuzzy sets within the Footprint of Uncertainty (FoU) of the type-2 fuzzy set (shaded in grey).
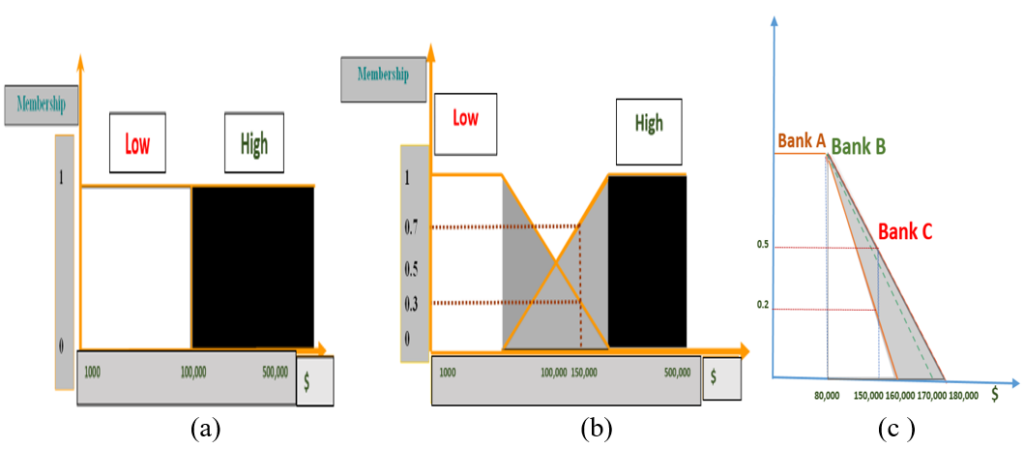
Figure 2. Representing the sets Low and High Annual Income using (a) Boolean sets. (b) Type-1 fuzzy sets. (c) Type-2 fuzzy sets for Low Income.
However, FLSs are not widely explored as an XAI technique and they do not appear in the analysis shown in Figure1a. One reason might be that FLSs are associated with control problems and they are not widely perceived as a ML tool. Logical Glue’s did produce novel patented systems which were able to use evolutionary systems to generate FLSs with short IF-Then rules and small rule bases while maximizing prediction accuracy –see – Figure 3a (getting closer to accuracies of black box models).
The type-2 FLSs generates IF-Then rules which get the data to speak the same language as humans. As shown in Figure 3b, this allows humans to easily analyze and interpret the generated models and augment such rule bases with rules which capture their expertise. They allow people to cover gaps in the data to provide a unique framework for integrating data-driven and expert knowledge. This allows the user to have full trust in the generated model and cover the XAI components related to Transparency, Causality, Bias, Fairness and Safety.
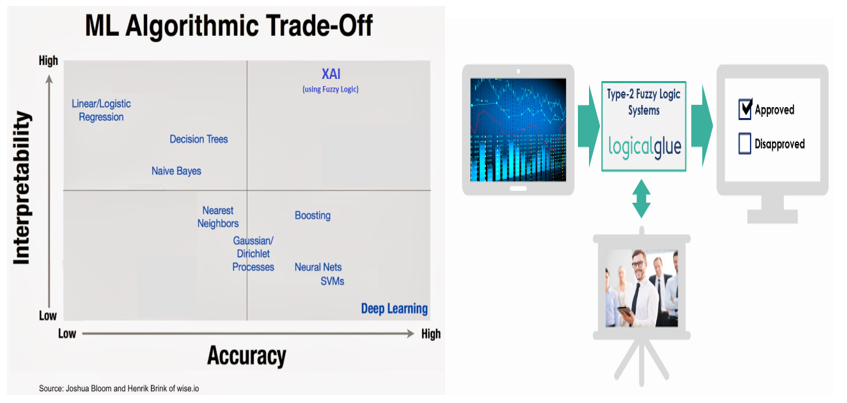
Figure (3): (a) Logicalglue XAI model striking a balance between accuracy and interpretability. (b) Logicalglue model providing the ability to integrate data driven and expert knowledge.